CASE STUDY
OmniAb Increases B Cell Analysis Throughput by 10x Using LandingLens
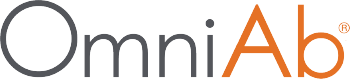
Bob Chen, PhD., Sr. Director of System Engineering for OmniAb, and his team are discovering innovative antibody therapeutics by using their xPloration® platform to capture images of millions of single B cell assays. This evaluation requires expert judgment through manual visual review by experts. By automating this manual process with LandingLens, Bob’s team was able to increase their inspection throughput by up to 10X and to find up to 30% more potential hits than by visual inspection.
“We are excited by the positive impact our partnership has generated. The ease of use of LandingLens has democratized AI to other parts of our company and has enabled us to use AI in additional use cases,” Bob said.
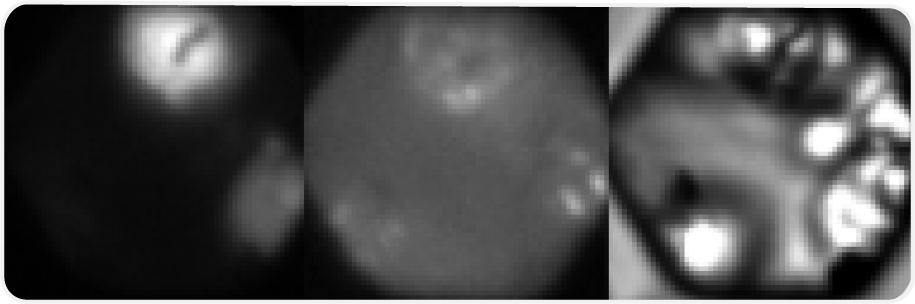
Unlabeled image
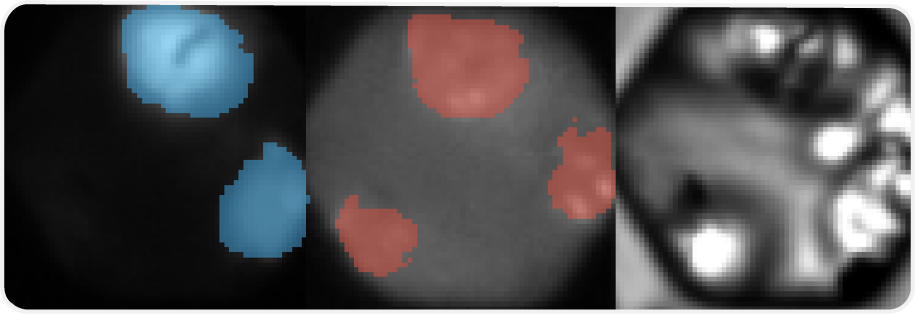
Prediction made by LandingAI
Customer Objectives
- Automate people-intensive visual inspection process
- Increase number of usable cells identified for further study to improve drug efficacy
Key results
- Increased throughput of visual inspection process by up to 10x
- Improved usable cell identification process by 30%
Bob Chen, Ph.D.
Sr. Director of Systems Engineering
OmniAb
Discovering Life-Saving Antibodies
OmniAb’s mission is to enable the rapid development of innovative therapeutics by pushing the frontiers of drug discovery technologies. They provide the world’s leading pharmaceutical companies with cutting-edge technologies, such as diverse antibody repertoires from transgenic animals and high-throughput screening technologies.
Manual, Multi-Faceted Process
OmniAb’s xPloration® screening platform uses automated microscopes to capture images of millions of B cells interacting with reporter cells or beads. These pictures are then analyzed to determine if individual B cells express antibodies having the desired function. Those desirable B cells are then efficiently recovered for further study.
The criteria for what qualifies for further study are multi-faceted and require expert judgment. These microscopic images are analyzed in multiple color channels, and the interactions between the antibodies and targeted cells are difficult to detect to untrained eyes since the appropriate interactions require analyzing overlapping color channels. This process requires manual review by scientists with PhDs who visually inspect these images and apply rules from their defect book to identify appropriate cells.
The manual visual inspection is fairly accurate in the beginning of an experiment. However, the review process would slow over time due to human fatigue and concern for cell health. Since the team works with live cells, they have limited inspection time. Often the team would cut the inspection short due to time limitations.
“It became obvious to me that these workflows could be significantly improved ,” said Bob. “There was also an element of human subjectivity that needed to be applied. What one person considers a valid specimen for further study, another person may not, which can produce inconsistent results.”
So Bob sought to bring the power of AI and automation to his team to further empower the OmniAb antibody discovery platforms.
Proving the Value of AI
After some initial success with internal AI systems, Bob evaluated LandingLens to improve this process. Once his team uploaded images into LandingLens, they appreciated using the product’s easy-to-use labeling tool. LandingLens’ data-centric approach allowed them to focus on getting consistent, high quality labeled images from the PhDs with domain knowledge to train a reliable model.
“Because of the throughput of our platform, we are able to image each of the millions of cells we screen. Even in highly complex campaigns, we find the rare samples. Out of a thousand images, we may only have maybe 10 images that have what we are looking for,” he said.
Due to the user-friendliness of the LandingLens platform, OmniAb is able to democratize the building of AI models to new roles such as hardware and software engineers, recent college graduates and even external consultants or contractors. These users are able to leverage state-of-the-art deep learning models to not only train but also iterate through the models with built-in visual model error analysis tools without having deep knowledge or expertise in AI and deep learning.
“Once you have a trustable model, you can democratize AI to other parts of the organization and to less experienced users,” said Bob. “We’ve been using LandingAI more and more for new use cases that involve people who are not high-level scientists because we have a reliable model that we know works.”
“It was important for us to partner with a company like LandingAI because they bring such a deep level of AI expertise to our unique high throughput use case. We view this partnership as synergistic with our cutting-edge tools and broad base of data in antibody discovery. Now we’re able to scale our operations in a grounded, proven way.”
Accelerating Throughput by 10X
OmniAb’s data and model pipelines were built using Python, and they concatenated multiple color images into a single image to train their model. Using LandingAI’s automated data augmentation features, they were able to train a state-of-the-art deep learning model without any hyperparameter tuning, achieving 96.8% for both precision* & recall* while maintaining a mIOU score of 0.83. They started iterating on the model with just 100 labeled images at first to prove the use case then gradually increased the number of images they labeled using LandingAI’s image labeling and reviewing task management features to scale their labeling process without compromising on quality, leading to their final, high-performing model.
LandingLens model versioning and automated performance metrics
For inference, they are using LandingAI’s edge computing software, Landing Edge. With its folder monitoring feature, they are able to run live inferences by importing the preprocessed images into the monitored folder from the data pipeline and extracting the prediction as a JSON file for each image. Then, the prediction is used to create a list of coordinates of all interesting microcapillaries and their machine recovers these cells via laser extraction. This entire process is now fully automated.
OmniAb’s xPloration Instrument for high throughput B cell screening
Get Started Using LandingLens In Your Application
Are you ready to see how LandingLens can improve your throughput? Get started for free.
1 Precision: Also known as positive predictive value. It helps describe the purity of the positive detections relative to the ground truth.
2 Recall: Also known as sensitivity. It helps describe the completeness of the positive predictions relative to the ground truth.
3 mIoU: Stands for “mean intersection over union”. It is the mean of the overlapping area between the predicted segmentation and the ground truth divided by the area of union between the predicted segmentation and the ground truth.